Mahdi Morafah
Machine Learning Researcher
Generative AI and Federated Learning Enthusiast
Former ML Research Intern at Tesla Autopilot and Qualcomm AI
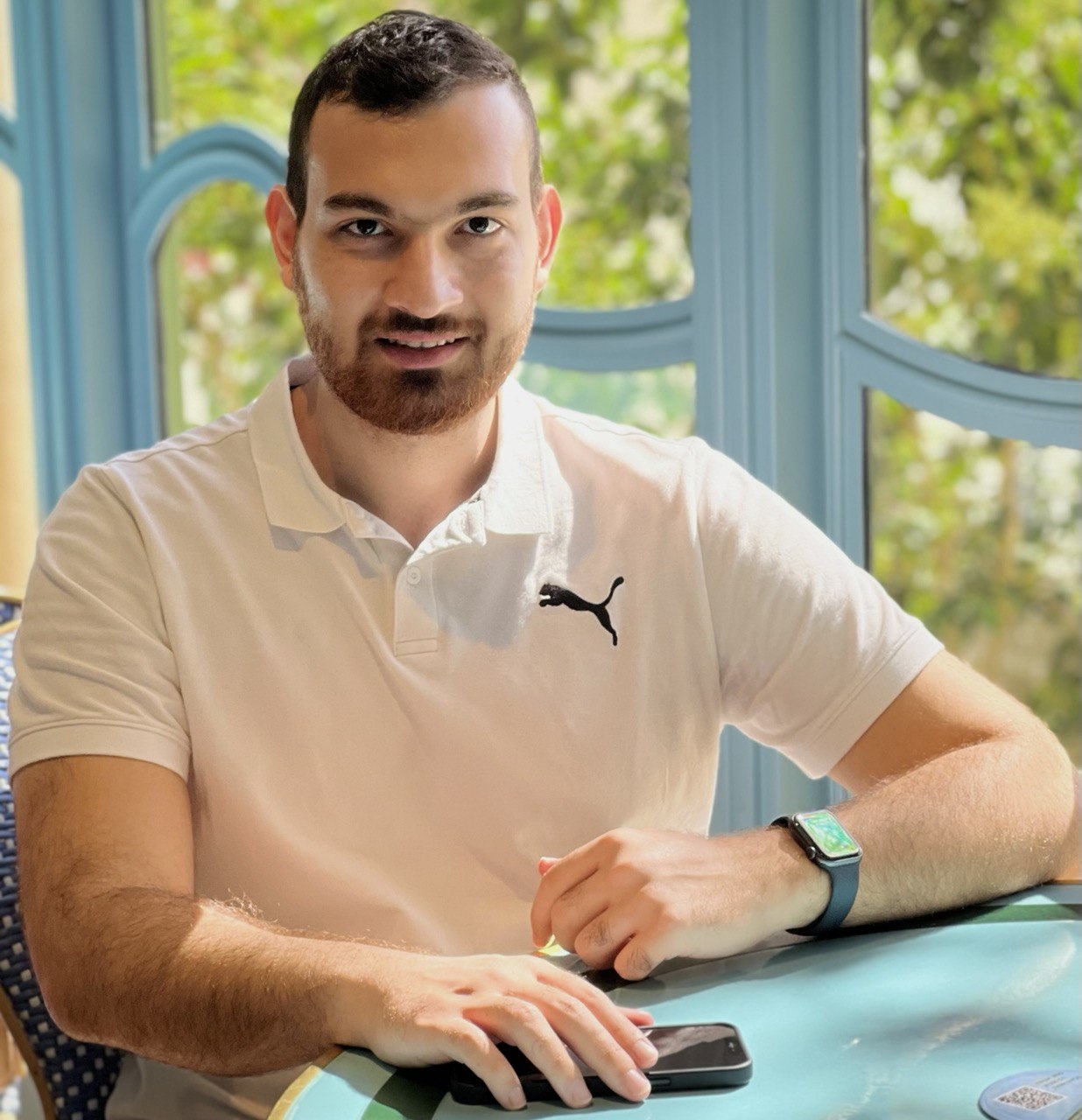
Department of Electrical and Computer Engineering
University of California San Diego
9500 Gilman Dr, La Jolla, CA 92093
Email: mmorafah 'at' ucsd 'dot' edu
I am a PhD Candidate (third year) in Electrical and Computer Engineering at University of California San Diego (UCSD), luckly being advised by Prof. Bill Lin.
I am broadly interested in Machine Learning and Optimization. My current research focus is on Federated Learning, Generative AI and Business. Here are the research themes that I am currently working on:
- Large Scale Federated Learning Over Diverse Heterogeneous Devices
- Efficient Training and Fine-tuning of Large Language Models in Federated Learning
- Fast Sampling for Diffusion Models
- Multi-Modal Foundation Models
- Effect of Generative AI in Business Market
I previously received a M.S. in Electrical and Computer Engineering at UCSD in 2021, and a B.S. in Electrical Engineering at Tehran Polytechnique.
I also working as a machine learning research intern at Tesla Autopilot and Qualcomm AI.
I am looking for machine learning internship positions for summer 2024.
I am open to collaborate on interesting project, please feel free to reach out.
news
Nov 20, 2023 | I am excited to successfully pass my PhD qualifying exam and being advanced to candidacy! |
---|---|
Jul 20, 2023 | New accepted paper “A Practical Recipe for Federated Learning Under Statistical Heterogeneity Experimental Design” [Accepted to IEEE Transactions on AI (Jul, 2023)]. |
May 31, 2023 | Served as Reviewer for IEEE TCNS and IEEE CDC. |
Jan 5, 2023 | Awarded AAAI 2023 student scholarship for conference attendance. |
Sep 30, 2022 | New paper on arXiv “Rethinking Data Heterogeneity in Federated Learning: Introducing a New Notion and Standard Benchmarks” [Accepted to FL NeurIPS’22 Workshops (Oct, 2022)]. |
Sep 21, 2022 | New paper on arXiv “Efficient Distribution Similarity Identification in Clustered Federated Learning via Principal Angles Between Client Data Subspaces” [Accepted to AAAI’23 (acceptance rate=19.6%) Nov, 2022]. |
selected publications
- IEEE OJCSarXiv preprint arXiv:2208.09754 2022
- IEEE TCNSarXiv preprint arXiv:2110.10406 2021
- IEEE TAIIEEE Transactions on Artificial Intelligence 2023